An artificial intelligence (AI) agent is a system that autonomously performs specific tasks for users or systems by planning complex workflows and using tools. They utilize the sophisticated natural language processing (NLP) methods of large language models (LLMs) to understand and respond to user inputs incrementally and decide when to use external tools. Beyond natural language processing (NLP), these agents handle the decision-making process, problem-solving, and external interactions. For instance, an AI agent managing e-commerce customer support can interpret queries, access databases, take actions like issuing refunds, and interact with APIs for real-time updates or logistics coordination, ensuring seamless follow-ups and updates.
So, an AI agent is like a helpful buddy who assists you by handling repetitive tasks, making decisions, and simplifying complex processes. It understands your requests, uses the tools and resources available, and gets the job done efficiently. That’s super cool, but how did we get here? That will require a bit of a dive into various artificial intelligence (AI) models and how they’ve changed over time to get to this point. Let’s get started.
How did we get here?
Artificial Intelligence (AI) has evolved significantly over time, moving from simple, rule-based systems to sophisticated, autonomous AI agents capable of handling complex tasks. Early AI relied on predefined instructions, or “control logic”, where every decision was explicitly programmed. These systems, often part of what we call compound AI systems, were modular and task-specific. For example, one module might identify objects in an image, while another might provide recommendations. While effective for narrowly defined problems, these systems lacked the flexibility to adapt or make informed decisions on their own.
The development of LLMs, such as OpenAI’s GPT series, has been a game-changer in AI. LLMs are advanced neural networks trained on massive amounts of text data, enabling them to understand and generate human-like text. While dating back to the 1980s, their use within the context of generative AI is a bit more modern. LLMs are at the heart of many AI agents because they allow the agent to comprehend user requests, decide on a course of action, and even call external tools when necessary.
To transition from compound AI systems to agentic AI systems, a shift in control logic was necessary. Instead of rigid, predefined workflows, agentic systems rely on dynamic control logic, enabling them to assess a situation, plan their actions, and execute decisions based on real-time data autonomously. You can think of this shift from compound to agentic control logic as putting the LLM in its own driver’s seat rather than the predefined instructions. This change allowed AI to move from being a collection of independent modules to a cohesive system that supports learning and adaptation, a “thinking” system capable of adapting to new challenges. By combining the reasoning capabilities of LLMs with this agentic control logic, modern AI agents can handle diverse and complex tasks. This brings a new level of intelligence and autonomy to how AI interacts with the world, especially in areas like software development and virtual assistants.
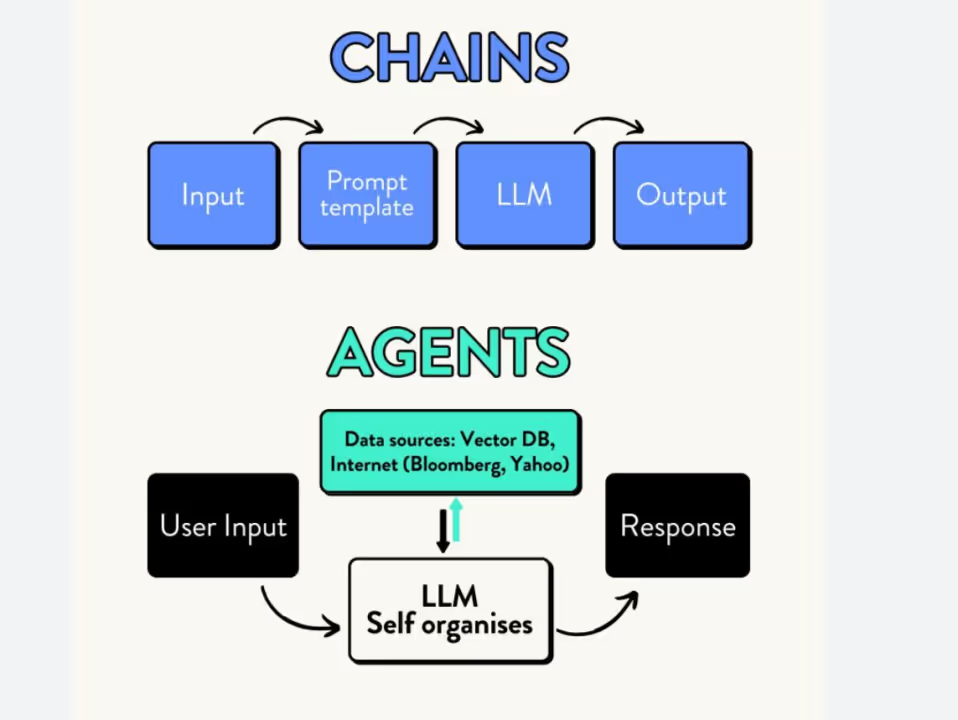
Why does it matter?
Alright. Now that we’ve learned about how we got here. The next question is, what’s the big deal? Why does it matter that agentic AI can govern itself, adapt, plan actions, and execute tasks with autonomous AI agents? These systems promise to revolutionize various domains by increasing efficiency, enabling new applications, and reducing the need for direct human intervention. However, they also raise questions about safety, accountability, and societal impact.
First, agentic AI boosts efficiency and productivity by solving complex problems with minimal supervision. In logistics, it optimizes global supply chains for cost and environmental benefits. In healthcare, it adapts to new data for real-time diagnostics and personalized treatments. It also enables breakthroughs in challenging environments like space exploration or deep-sea missions, where autonomous systems make decisions in real-time, such as driving cars and Mars rovers navigating and analyzing terrain. Additionally, agentic AI personalizes solutions, allowing for tasks such as customizing education plans based on student progress or optimizing financial portfolios in dynamic markets. These benefits stem from the ability of agentic systems to make informed decisions through their understanding of context and goals.
The societal impacts of agentic AI are profound. By enabling new business models, reducing costs, and improving accessibility, these systems can reshape industries and democratize resources. For example, remote areas could gain access to high-quality education or healthcare through autonomous platforms designed to operate independently of centralized infrastructure. However, this potential is accompanied by significant challenges that must be addressed.
Safety and alignment are key concerns for agentic AI. Ensuring these systems align with human values is critical, as misaligned objectives could lead to harmful outcomes. For instance, an autonomous trading system destabilizes markets by prioritizing profits over ethics. Another issue is AI errors or hallucinations, where models generate incorrect outputs due to flawed data or misinterpretation. This showcases the need for human oversight of AI models to maintain accuracy. And what about when an AI agent makes a harmful decision? It is unclear who should be held responsible: the developers, the operators, or the AI itself? This ambiguity complicates the ethical and legal frameworks required to govern such systems. Autonomous systems also challenge traditional notions of control. While their independence is a strength, it is essential to design mechanisms that allow human oversight and intervention to prevent misuse or failure.
Agentic AI also carries significant economic and social implications. While it creates opportunities, it may displace jobs, widen inequalities, and concentrate power among a few entities. For instance, widespread adoption in manufacturing or logistics could eliminate millions of jobs, necessitating policies to manage disruption and distribute benefits fairly. Additionally, many prefer human interaction, as seen in AI-led call centers where customers often demand to “speak to a real person”. This lack of human interaction can drastically reduce customer satisfaction and efficiency if the AI agent cannot give the customer what they want. This supports arguments for AI assisting rather than replacing humans. Security concerns also loom, as agentic AI could be exploited for cyberattacks or autonomous weapons. All of this raises urgent questions about the regulation and governance of these technologies to prevent misuse.

What’s next?
So now we know what AI agents are, why they’re important, what they can do, and some of the risks associated with them. Where do we go from here? Well, to implement and utilize AI agents effectively while mitigating their potential risks, we need a comprehensive approach that balances innovation with safety, ethical considerations, and governance. This will require collaboration between governments, industry leaders, researchers, developers, and society to create frameworks that guide the development and deployment of advanced AI systems responsibly.
To ensure safe and ethical use of agentic AI, clear regulatory frameworks are vital. Governments and international bodies must establish guidelines addressing data privacy, algorithmic bias, and decision explainability. For instance, rigorous testing and certification for autonomous vehicles can prevent accidents and build trust. Embedding ethical principles in AI design is equally important. Practices like value alignment and involving diverse perspectives, from those such as ethicists, sociologists, and community representatives, help ensure systems meet specific goals and societal needs. For example, creating AI systems for healthcare with input from patients, clinicians, and advocacy groups can help ensure they are equitable and effective.
Oversight mechanisms are also critical. This includes “kill switches” for halting harmful AI behavior and independent review boards to audit high-risk systems for compliance with safety and ethics. For example, an AI agent used for managing critical infrastructure, such as power grids, should undergo periodic assessments to verify its reliability and alignment with human oversight. Encouraging innovation through public-private partnerships can also drive the development of safe, impactful AI systems while providing oversight to ensure alignment with societal needs.
Finally, building public awareness and trust is essential for the successful adoption of agentic AI. Educating the public about the benefits, limitations, and risks of these systems can help manage expectations and foster acceptance. Transparent communication about how AI systems work and the guardrails in place to prevent harm is key to gaining user confidence.
The rise of agentic AI represents a transformative milestone in artificial intelligence, with the potential to revolutionize industries, enhance efficiency, and open new frontiers. Yet, its autonomy also brings challenges in safety, accountability, ethics, and societal impact. To harness its benefits while minimizing risks, we need a balanced approach focused on enhancing human well-being, upholding ethical standards, and fostering equity and sustainability. This requires clear regulations, ethical design, human oversight, and transparency, alongside efforts to educate the public. Moving forward, collaboration and a long-term commitment to societal interests must guide the development of these powerful technologies. The journey toward responsible and impactful AI agents is not without challenges, but it offers an unprecedented opportunity to reshape our world for the better. By prioritizing innovation alongside safety and ethical governance, we can harness the full potential of AI agents to address some of society’s most pressing challenges and contribute to a more equitable, sustainable, and prosperous future.
References
Dahmoun, Miriam. “The Rise of Agentic AI: Revolutionizing Autonomy and Intelligence in the Digital Age.” LinkedIn, LinkedIn, 21 Oct. 2024, www.linkedin.com/pulse/rise-agentic-ai-revolutionizing-autonomy-intelligence-miriam-hw7fc/.
Gutowska, Anna. “What Are AI Agents?” IBM, IBM, 3 July 2024, www.ibm.com/think/topics/ai-agents.
“Here’s How AI Is Changing NASA’s Mars Rover Science.” NASA Jet Propulsion Laboratory, NASA, 16 July 2024, www.jpl.nasa.gov/news/heres-how-ai-is-changing-nasas-mars-rover-science/.
Kass-Hout, Taha, and Dan Sheeran. “How Agentic AI Systems Can Solve the Three Most Pressing Problems in Healthcare Today.” GE HealthCare, GE HealthCare, 10 Dec. 2024, aws.amazon.com/blogs/industries/how-agentic-ai-systems-can-solve-the-three-most-pressing-problems-in-healthcare-today/.
Kaufman, Stephen. “Beyond ChatGPT: The Rise of Agentic AI and Its Implications for Security.” CSO, IDG Communications, Inc., 22 Oct. 2024, www.csoonline.com/article/3574697/beyond-chatgpt-the-rise-of-agentic-ai-and-its-implications-for-security.html.
Litan, Avivah. “Gartner: Mitigating Security Threats in AI Agents: Computer Weekly.” ComputerWeekly.Com, Informa TechTarget, 23 Sept. 2024, www.computerweekly.com/opinion/Gartner-Mitigating-security-threats-in-AI-agents.
Srivatsa, Harsha. “Unleashing Generative AI’s Potential: LLM Chains, Agentic AI, and the Future of AI Product Architecture.” LinkedIn, 27 July 2024, www.linkedin.com/pulse/unleashing-generative-ais-potential-llm-chains-agentic-srivatsa-xl7nc/.
Varghese, Benita Elizabeth. “Mitigating Agentic AI Risks | The Critical Role of Guardrails.” SearchUnify, SearchUnify, 21 Oct. 2024, www.searchunify.com/blog/mitigating-agentic-ai-risks-the-critical-role-of-guardrails/#:~:text=Agent%20Helper%2C%20as%20an%20agentic,or%20violations%20of%20ethical%20guidelines.
“What Is Agentic AI? Redefining Business Process Automation with Autonomous Agents.” DRUID, DRUID, 18 Nov. 2024, www.druidai.com/blog/agentic-ai-redefining-process-automation-with-autonomous-agents.
About the Author
Grace Dees is the Cybersecurity Business Analyst at Resonance Security. She specializes in the intersection of traditional and Web3 security by bridging the gap between technology and business objectives to deliver impactful solutions aligned with client needs.